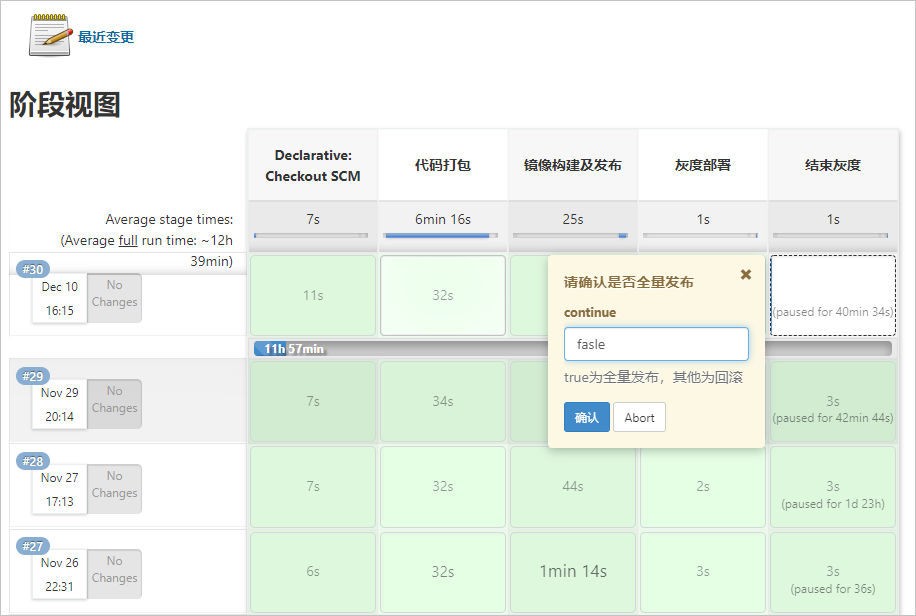
Hello Halo
如果你看到了这一篇文章,那么证明你已经安装成功了,感谢使用 Halo 进行创作,希望能够使用愉快。
相关链接
在使用过程中,有任何问题都可以通过以上链接找寻答案,或者联系我们。
这是一篇自动生成的文章,请删除这篇文章之后开始你的创作吧!
Producer 数据发送流程
下面通过对 send
源码分析来一步步剖析 Producer 数据的发送流程。
Producer 的 send 实现
用户是直接使用 producer.send()
发送的数据,先看一下 send()
接口的实现
// 异步向一个 topic 发送数据
@Override
public Future<RecordMetadata> send(ProducerRecord<K, V> record) {
return send(record, null);
}
// 向 topic 异步地发送数据,当发送确认后唤起回调函数
@Override
public Future<RecordMetadata> send(ProducerRecord<K, V> record, Callback callback) {
// intercept the record, which can be potentially modified; this method does not throw exceptions
ProducerRecord<K, V> interceptedRecord = this.interceptors == null ? record : this.interceptors.onSend(record);
return doSend(interceptedRecord, callback);
}
数据发送的最终实现还是调用了 Producer 的 doSend()
接口。
Producer 的 doSend 实现
下面是 doSend()
的具体实现
private Future<RecordMetadata> doSend(ProducerRecord<K, V> record, Callback callback) {
TopicPartition tp = null;
try {
// 1.确认数据要发送到的 topic 的 metadata 是可用的
ClusterAndWaitTime clusterAndWaitTime = waitOnMetadata(record.topic(), record.partition(), maxBlockTimeMs);
long remainingWaitMs = Math.max(0, maxBlockTimeMs - clusterAndWaitTime.waitedOnMetadataMs);
Cluster cluster = clusterAndWaitTime.cluster;
// 2.序列化 record 的 key 和 value
byte[] serializedKey;
try {
serializedKey = keySerializer.serialize(record.topic(), record.key());
} catch (ClassCastException cce) {
throw new SerializationException("Can't convert key of class " + record.key().getClass().getName() +
" to class " + producerConfig.getClass(ProducerConfig.KEY_SERIALIZER_CLASS_CONFIG).getName() +
" specified in key.serializer");
}
byte[] serializedValue;
try {
serializedValue = valueSerializer.serialize(record.topic(), record.value());
} catch (ClassCastException cce) {
throw new SerializationException("Can't convert value of class " + record.value().getClass().getName() +
" to class " + producerConfig.getClass(ProducerConfig.VALUE_SERIALIZER_CLASS_CONFIG).getName() +
" specified in value.serializer");
}
// 3. 获取该 record 的 partition 的值(可以指定,也可以根据算法计算)
int partition = partition(record, serializedKey, serializedValue, cluster);
int serializedSize = Records.LOG_OVERHEAD + Record.recordSize(serializedKey, serializedValue);
ensureValidRecordSize(serializedSize); // record 的字节超出限制或大于内存限制时,就会抛出 RecordTooLargeException 异常
tp = new TopicPartition(record.topic(), partition);
long timestamp = record.timestamp() == null ? time.milliseconds() : record.timestamp(); // 时间戳
log.trace("Sending record {} with callback {} to topic {} partition {}", record, callback, record.topic(), partition);
Callback interceptCallback = this.interceptors == null ? callback : new InterceptorCallback<>(callback, this.interceptors, tp);
// 4. 向 accumulator 中追加数据
RecordAccumulator.RecordAppendResult result = accumulator.append(tp, timestamp, serializedKey, serializedValue, interceptCallback, remainingWaitMs);
// 5. 如果 batch 已经满了,唤醒 sender 线程发送数据
if (result.batchIsFull || result.newBatchCreated) {
log.trace("Waking up the sender since topic {} partition {} is either full or getting a new batch", record.topic(), partition);
this.sender.wakeup();
}
return result.future;
} catch (ApiException e) {
log.debug("Exception occurred during message send:", e);
if (callback != null)
callback.onCompletion(null, e);
this.errors.record();
if (this.interceptors != null)
this.interceptors.onSendError(record, tp, e);
return new FutureFailure(e);
} catch (InterruptedException e) {
this.errors.record();
if (this.interceptors != null)
this.interceptors.onSendError(record, tp, e);
throw new InterruptException(e);
} catch (BufferExhaustedException e) {
this.errors.record();
this.metrics.sensor("buffer-exhausted-records").record();
if (this.interceptors != null)
this.interceptors.onSendError(record, tp, e);
throw e;
} catch (KafkaException e) {
this.errors.record();
if (this.interceptors != null)
this.interceptors.onSendError(record, tp, e);
throw e;
} catch (Exception e) {
if (this.interceptors != null)
this.interceptors.onSendError(record, tp, e);
throw e;
}
}
在 dosend()
方法的实现上,一条 Record 数据的发送,可以分为以下五步:
确认数据要发送到的 topic 的 metadata 是可用的(如果该 partition 的 leader 存在则是可用的,如果开启权限时,client 有相应的权限),如果没有 topic 的 metadata 信息,就需要获取相应的 metadata;
序列化 record 的 key 和 value;
获取该 record 要发送到的 partition(可以指定,也可以根据算法计算);
向 accumulator 中追加 record 数据,数据会先进行缓存;
如果追加完数据后,对应的 RecordBatch 已经达到了 batch.size 的大小(或者batch 的剩余空间不足以添加下一条 Record),则唤醒
sender
线程发送数据。
数据的发送过程,可以简单总结为以上五点,下面会这几部分的具体实现进行详细分析。
发送过程详解
获取 topic 的 metadata 信息
Producer 通过 waitOnMetadata()
方法来获取对应 topic 的 metadata 信息,这部分后面会单独抽出一篇文章来介绍,这里就不再详述,总结起来就是:在数据发送前,需要先该 topic 是可用的。
key 和 value 的序列化
Producer 端对 record 的 key
和 value
值进行序列化操作,在 Consumer 端再进行相应的反序列化,Kafka 内部提供的序列化和反序列化算法如下图所示:
当然我们也是可以自定义序列化的具体实现,不过一般情况下,Kafka 内部提供的这些方法已经足够使用。
获取 partition 值
关于 partition 值的计算,分为三种情况:
指明 partition 的情况下,直接将指明的值直接作为 partiton 值;
没有指明 partition 值但有 key 的情况下,将 key 的 hash 值与 topic 的 partition 数进行取余得到 partition 值;
既没有 partition 值又没有 key 值的情况下,第一次调用时随机生成一个整数(后面每次调用在这个整数上自增),将这个值与 topic 可用的 partition 总数取余得到 partition 值,也就是常说的
round-robin
算法。
具体实现如下:
// 当 record 中有 partition 值时,直接返回,没有的情况下调用 partitioner 的类的 partition 方法去计算(KafkaProducer.class)
private int partition(ProducerRecord<K, V> record, byte[] serializedKey, byte[] serializedValue, Cluster cluster) {
Integer partition = record.partition();
return partition != null ?
partition :
partitioner.partition(
record.topic(), record.key(), serializedKey, record.value(), serializedValue, cluster);
}
Producer 默认使用的 partitioner
是 org.apache.kafka.clients.producer.internals.DefaultPartitioner
,用户也可以自定义 partition 的策略,下面是这个类两个方法的具体实现:
public int partition(String topic, Object key, byte[] keyBytes, Object value, byte[] valueBytes, Cluster cluster) {
List<PartitionInfo> partitions = cluster.partitionsForTopic(topic);
int numPartitions = partitions.size();
if (keyBytes == null) {// 没有指定 key 的情况下
int nextValue = nextValue(topic); // 第一次的时候产生一个随机整数,后面每次调用在之前的基础上自增;
List<PartitionInfo> availablePartitions = cluster.availablePartitionsForTopic(topic);
// leader 不为 null,即为可用的 partition
if (availablePartitions.size() > 0) {
int part = Utils.toPositive(nextValue) % availablePartitions.size();
return availablePartitions.get(part).partition();
} else {
return Utils.toPositive(nextValue) % numPartitions;
}
} else {// 有 key 的情况下,使用 key 的 hash 值进行计算
return Utils.toPositive(Utils.murmur2(keyBytes)) % numPartitions; // 选择 key 的 hash 值
}
}
// 根据 topic 获取对应的整数变量
private int nextValue(String topic) {
AtomicInteger counter = topicCounterMap.get(topic);
if (null == counter) { // 第一次调用时,随机产生
counter = new AtomicInteger(new Random().nextInt());
AtomicInteger currentCounter = topicCounterMap.putIfAbsent(topic, counter);
if (currentCounter != null) {
counter = currentCounter;
}
}
return counter.getAndIncrement(); // 后面再调用时,根据之前的结果自增
}
这就是 Producer 中默认的 partitioner 实现。
向 accumulator 写数据
Producer 会先将 record 写入到 buffer 中,当达到一个 batch.size
的大小时,再唤起 sender
线程去发送 RecordBatch
(第五步),这里先详细分析一下 Producer 是如何向 buffer 中写入数据的。
Producer 是通过 RecordAccumulator
实例追加数据,RecordAccumulator
模型如下图所示,一个重要的变量就是 ConcurrentMap<TopicPartition, Deque<RecordBatch>> batches
,每个 TopicPartition
都会对应一个 Deque<RecordBatch>
,当添加数据时,会向其 topic-partition 对应的这个 queue 最新创建的一个 RecordBatch
中添加 record,而发送数据时,则会先从 queue 中最老的那个 RecordBatch
开始发送。
// org.apache.kafka.clients.producer.internals.RecordAccumulator
// 向 accumulator 添加一条 record,并返回添加后的结果(结果主要包含: future metadata、batch 是否满的标志以及新 batch 是否创建)其中, maxTimeToBlock 是 buffer.memory 的 block 的最大时间
public RecordAppendResult append(TopicPartition tp,
long timestamp,
byte[] key,
byte[] value,
Callback callback,
long maxTimeToBlock) throws InterruptedException {
appendsInProgress.incrementAndGet();
try {
Deque<RecordBatch> dq = getOrCreateDeque(tp);// 每个 topicPartition 对应一个 queue
synchronized (dq) {// 在对一个 queue 进行操作时,会保证线程安全
if (closed)
throw new IllegalStateException("Cannot send after the producer is closed.");
RecordAppendResult appendResult = tryAppend(timestamp, key, value, callback, dq); // 追加数据
if (appendResult != null)// 这个 topic-partition 已经有记录了
return appendResult;
}
// 为 topic-partition 创建一个新的 RecordBatch, 需要初始化相应的 RecordBatch,要为其分配的大小是: max(batch.size, 加上头文件的本条消息的大小)
int size = Math.max(this.batchSize, Records.LOG_OVERHEAD + Record.recordSize(key, value));
log.trace("Allocating a new {} byte message buffer for topic {} partition {}", size, tp.topic(), tp.partition());
ByteBuffer buffer = free.allocate(size, maxTimeToBlock);// 给这个 RecordBatch 初始化一个 buffer
synchronized (dq) {
if (closed)
throw new IllegalStateException("Cannot send after the producer is closed.");
RecordAppendResult appendResult = tryAppend(timestamp, key, value, callback, dq);
if (appendResult != null) {// 如果突然发现这个 queue 已经存在,那么就释放这个已经分配的空间
free.deallocate(buffer);
return appendResult;
}
// 给 topic-partition 创建一个 RecordBatch
MemoryRecordsBuilder recordsBuilder = MemoryRecords.builder(buffer, compression, TimestampType.CREATE_TIME, this.batchSize);
RecordBatch batch = new RecordBatch(tp, recordsBuilder, time.milliseconds());
// 向新的 RecordBatch 中追加数据
FutureRecordMetadata future = Utils.notNull(batch.tryAppend(timestamp, key, value, callback, time.milliseconds()));
dq.addLast(batch);// 将 RecordBatch 添加到对应的 queue 中
incomplete.add(batch);// 向未 ack 的 batch 集合添加这个 batch
// 如果 dp.size()>1 就证明这个 queue 有一个 batch 是可以发送了
return new RecordAppendResult(future, dq.size() > 1 || batch.isFull(), true);
}
} finally {
appendsInProgress.decrementAndGet();
}
}